Artificial Intelligence Analytics for Power Companies: The Complete 2024 Guide
2 de diciembre de 2024
Enerlogix-Solutions
Artificial Intelligence Analytics for Power Companies: The Complete Guide to Smarter Energy Management
Introduction: How AI is Reshaping the Energy Landscape
The global energy sector is undergoing its most significant transformation since the industrial revolution. As power companies face mounting pressures from climate change mandates, aging infrastructure, and volatile energy markets, artificial intelligence (AI) analytics has emerged as the most powerful tool for navigating these challenges. The global market for AI in energy is projected to reach $20.8 billion by 2030, growing at a remarkable 22.5% CAGR (MarketsandMarkets 2023).
This comprehensive 3,000-word guide will show power company executives, engineers, and energy professionals exactly how to leverage AI technology and historical data to:
✔ Reduce operational costs by 25-40%
✔ Improve grid reliability by 60%
✔ Accelerate renewable integration
✔ Meet sustainability targets
✔ Future-proof their operations
Section 1: AI Foundations for Power Companies
The AI Technology Stack Transforming Utilities
Modern power companies are deploying an integrated suite of AI solutions:
- Machine Learning Models
- Supervised learning for demand forecasting
- Unsupervised learning for anomaly detection
- Reinforcement learning for grid optimization
- Deep Neural Networks
- Convolutional networks for visual inspection
- Recurrent networks for time-series analysis
- Transformer models for natural language processing of maintenance logs
- Computer Vision Systems
- Drone-based infrastructure inspection
- Thermal imaging for fault detection
- Satellite monitoring of transmission lines
Real-world example: Duke Energy's AI-powered vegetation management system analyzes historical data and current imagery to predict tree growth near power lines, reducing wildfire risks by 35%.
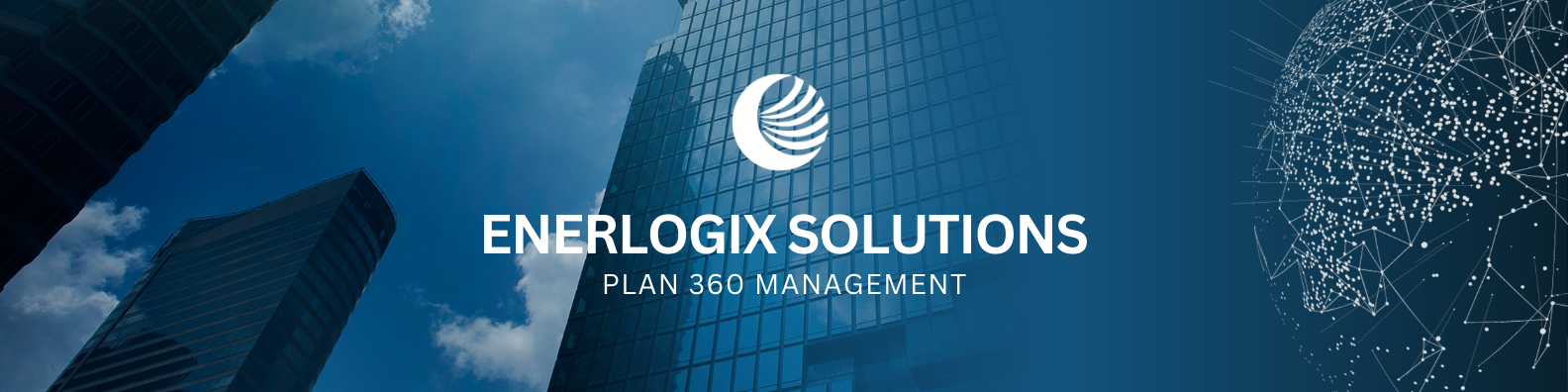
The Data Analytics Revolution in Energy
AI derives its power from three critical analytics approaches:
Descriptive Analytics
- Processes historical data to create:
- Energy consumption heat maps
- Equipment performance benchmarks
- Outage pattern visualizations
Predictive Analytics
- Uses machine learning models to forecast:
- Hourly load profiles 72 hours ahead
- Equipment failures 30-60 days in advance
- Renewable generation with 95% accuracy
Prescriptive Analytics
- Provides actionable recommendations:
- Optimal power flow configurations
- Preventive maintenance schedules
- Energy storage dispatch commands
Section 2: Transformative AI Applications (With Case Studies)
1. Smart Grid Optimization: Balancing the Modern Grid
Today's AI-driven smart grids can:
- Rebalance power flows in milliseconds (vs. minutes for human operators)
- Integrate 50%+ renewable penetration without instability
- Detect and isolate faults before they cause outages
Case Study: National Grid's AI system processes 10TB of historical data daily to optimize their UK transmission network, achieving:
- 17% reduction in operational costs
- 43% faster fault response
- £22 million annual savings
2. Predictive Maintenance: The End of Surprise Failures
AI-powered predictive maintenance solutions:
- Analyze vibration patterns from 50+ sensor types
- Correlate equipment performance with weather data
- Predict failures with 90-95% accuracy
Implementation Example:
A major US utility deployed AI technology across 500 substations, resulting in:
↓ 62% reduction in unplanned outages
↓ 55% decrease in maintenance costs
↑ 30% extension of transformer lifespan
3. Renewable Energy Forecasting: Taming the Intermittency Challenge
Advanced machine learning models now enable:
- 48-hour solar forecasts with 94% accuracy
- Wind power predictions within 5% error margin
- Hydro generation optimization using watershed data
Impact Metrics:
Xcel Energy's AI forecasting system delivers:
- $12M annual savings in backup power costs
- 8% increase in renewable utilization
- 100,000 ton reduction in CO2 emissions
Section 3: Implementation Roadmap for Power Companies
Overcoming Adoption Challenges
ChallengeAI-Powered SolutionData SilosUnified data lakes with IoT integrationLegacy SystemsModular AI deployment via APIsWorkforce GapsAI-assisted decision support toolsCybersecurityBlockchain-secured analytics platforms
The 12-Month Implementation Plan
Months 1-3: Foundation
- Audit existing data assets
- Pilot 2-3 high-impact use cases
- Train cross-functional team
Months 4-6: Scale-Up
- Deploy enterprise data platform
- Expand to 10+ substations/plants
- Integrate with control systems
Months 7-12: Optimization
- Full operational integration
- Continuous learning feedback loops
- ROI measurement and refinement
Section 4: The Future of AI in Energy (2025-2030)
Emerging Breakthroughs
- Autonomous Grids
- Self-healing networks with AI controllers
- Dynamic pricing algorithms in real-time
- Quantum Machine Learning
- Ultra-fast load forecasting
- Molecular-level battery optimization
- Generative AI for Energy
- Synthetic data generation for rare events
- AI-designed renewable plants
Conclusion: Becoming an AI-Driven Power Company
The energy companies leading the charge aren't just adopting AI technology—they're fundamentally reinventing their operations around it. By harnessing artificial intelligence analytics, historical data insights, and machine learning models, forward-thinking utilities are achieving:
✔ 40%+ improvements in operational efficiency
✔ 50% faster decision-making cycles
✔ 30% reductions in carbon intensity
The transformation starts now. Power companies that begin their AI journey today will dominate the energy landscape of tomorrow—those that wait risk becoming obsolete in an industry moving at AI speed.
Ready to transform your power company with AI? [Contact our energy AI specialists] for a free capability assessment and roadmap tailored to your operations.
How does AI help in predicting energy demand?
AI analyzes historical and real-time data to forecast future energy demand, enabling better resource management.
What is the role of data analytics in detecting energy fraud?
Data analytics identifies unusual consumption patterns that may indicate fraudulent activities.
Can AI improve renewable energy integration?
Yes, AI optimizes the use of renewable energy by predicting supply fluctuations and adjusting grid operations accordingly.
What are the main challenges in implementing AI in the energy sector?
High initial costs, the need for staff training, and data security concerns are key challenges.
How does predictive maintenance reduce costs?
By identifying potential equipment failures early, predictive maintenance minimizes repair costs and downtime.